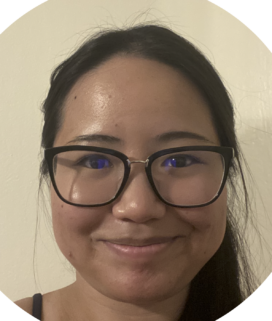
TITLE: “scDEED: a statistical method for detecting dubious 2D single-cell embeddings and optimizing t-SNE and UMAP hyperparameters.”
ABSTRACT: Two-dimensional (2D) embedding methods are crucial for single-cell data visualization. Popular methods such as t-distributed stochastic neighbor embedding(t-SNE) and uniform manifold approximation and projection (UMAP) are commonly used for visualizing cell clusters; however, it is well known that t-SNE and UMAP’s 2D embedding might not reliably inform the similarities among cell clusters. Motivated by this challenge, we present a statistical method, scDEED, for detecting dubious cell embeddings output by any 2D-embedding method. By calculating a reliability score for every cell embedding, scDEED identifies the cell embeddings with low reliability scores as dubious and those with high reliability scores as trustworthy. Moreover, by minimizing the number of dubious cell embeddings, scDEED provides intuitive guidance for optimizing the hyperparameters of an embedding method.