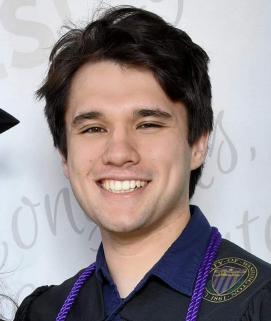
TITLE: “Tensor Factorization for Interpreting the Mechanisms of MRSA Persistence.”
ABSTRACT:
Methicillin-resistant Staphylococcus aureus (MRSA) bacteria is an increasingly common and life-threatening infection. While some antibiotics resolve MRSA infections in vitro, these same antibiotics often fail to clear an infection when used to treat patients, suggesting that MRSA persistence is a confluence of both host and bacterial factors. While recent research has identified critical genetic and proteomic determinants of MRSA persistence, the mechanisms that drive MRSA persistence are still poorly understood. Here, to better understand these mechanisms, we implement tensor-based factorization to integrate genetic and proteomic data collected from two cohorts of patients with MRSA infections. We find that our factorization process identifies patterns across biological modalities and is able to explain 75% of the variance observed in genetic and proteomic data with just 8 components. Additionally, this data integration improves persistence prediction accuracy as prediction models trained on these integrated factors demonstrate accuracies as high as 80% over subsets of the cohorts. Interpretation of these components further reveals mechanisms that drive the infection response and highlight processes critical for MRSA persistence. Overall, these results suggest that tensor-based factorization can identify the mechanisms underlying MRSA persistence across host factors and improve our ability to predict and understand MRSA persistence.